Ultrasound and microbubble contrast agents offer imaging and quantifying microcirculation / perfusion within the myocardium. Perfusion defects are an early indicator of coronary heart diseases as opposed to the current clinical practice which still relies on detection of cardiac wall motion abnormalities.
We are interested in developing novel imaging (e.g. high frame rate imaging) and image analysis techniques to image and quantify myocardium perfusion defects, in order for improved detection and treatment monitoring of coronary heart diseases.
Example projects:
High Frame-rate imaging of myocardium perfusion
There is currently significant interest in cardiac imaging with ultrasound (US) and new ultrafast techniques for increased frame rate using diverging transmission. However there has been to date no specific investigation reported, as far as we are aware, for Contrast Enhanced Ultrasound (CEUS) cardiac imaging using ultrafast ultrasound and diverging waves.
We have developed a High Frame Rate contrast Echocardiography (HFR CE) diverging wave imaging system using a cardiac probe and pulse inversion, and demonstrate its feasibility for heart perfusion applications [1]. The technique has been first evaluated on a simple test phantom in the laboratory [2] then on sheep model [3] and finally on healthy human volunteers [1].
The video below shows the conventional focus CE acquisition versus the HFR CE method proposed. Focus-CE is acquired with a frame rate of 25 frames per seconds while HFR-CE is acquired at 250 frames per seconds.
The Figure 1 shows the replenishment time-intensity curve of conventional focus CE and HFR CE acquisition after a flash destruction at time 0 seconds. It shows the possibility to use the Verasonics Platform to perform destruction-replenishment imaging at high frame rates.
Figure 1: Replenishment time-intensity curves in healthy human volunteers [1]
Thanks to the high frame rate acquisition, the flow velocity map within the cardiac chambers is successfully obtained as shown in Figure 2. The flow velocity map is obtained by using the HFR-CE transmission and our ultrasound imaging velocimetry (UIV) methods developed in the lab [4].
Figure 2: Chamber flow velocity map at different cardiac phases before and after R wave (R-0.12s, R-0.02s and R+0.12s respectively) [1]
Computer-Aided Perfusion Quantification in Myocardial Contrast Echocardiography
The assessment of blood perfusion in the microvessels of the heart is important in cardiology, especially for the diagnosis of coronary heart diseases (CAD). Myocardial contrast echocardiography (MCE) is one non-invasive way of assessing myocardial perfusion and uses the microbubbles as contrast agents. It is based on the bubble destruction and replenishment method (Figure 1). As MCE is noisy and highly variable, human visual assessments are subjective and unreliable. The project aims to develop novel semi-automatic computer-assisted tools and quantification methods (video, below) to improve the accuracy and reproducibility of myocardial perfusion quantification using MCE [5].
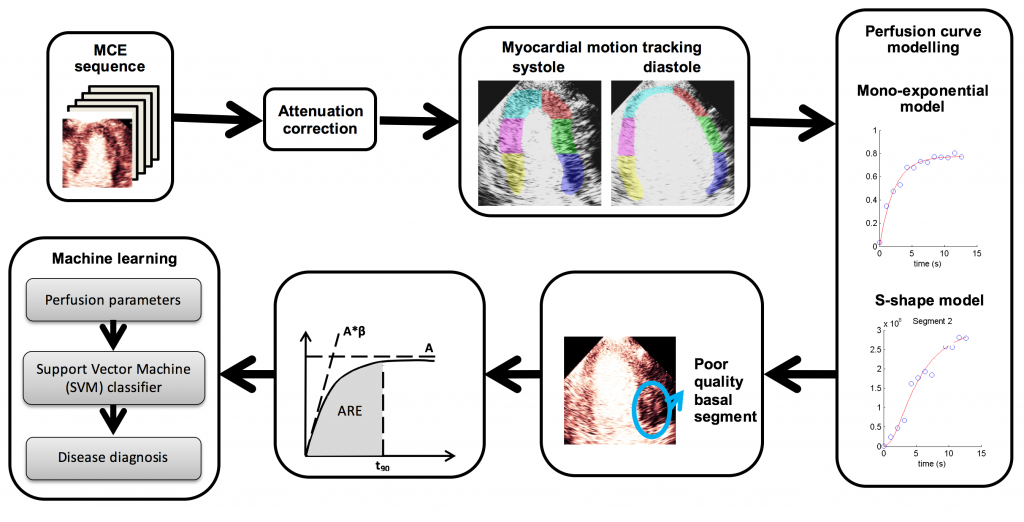
[vimeo]https://vimeo.com/156830372[/vimeo]
A real-time MCE sequence of the left ventricle showing perfusion in the myocardium. Tracking of the myocardium (red contour) is done by image registration.
Automatic Myocardium Segmentation in MCE
Myocardial contrast echocardiography (MCE) shows great potential for early detection and diagnosis of coronary artery diseases. But its quantitative analysis requires accurate myocardium segmentation. This is challenging due to the large sources of variations and noise found in MCE data. We aim to develop semi or fully automatic myocardium segmentation algorithms that are specifically designed for MCE data. Our work has focused on using random forest (Figure 3), statistical shape modelling (Figure 4) and image registration (video, above) to solve the segmentation problem [6].
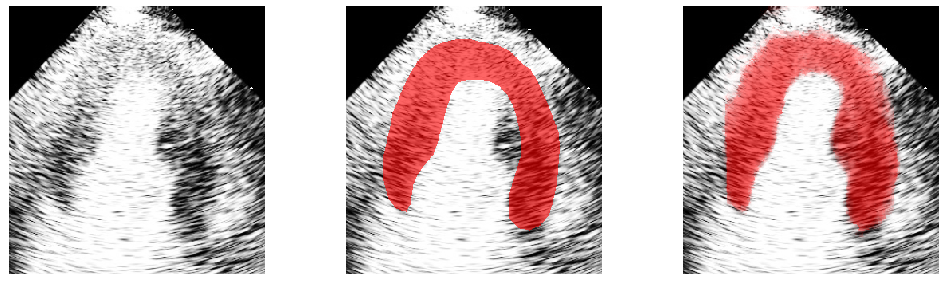

References
- M. Toulemonde, R. Corbett, V. Papadopoulou, N. Chahal, Y. Li, C. H. Leow, D. O. Cosgrove, R. J. Eckersley,N. Duncan, R. Senior, and M.X. Tang, “High Frame Rate Contrast Echocardiography – in human demonstration”, JACC Cardiovascular Imaging
- M Toulemonde, V Papadopoulou, MX Tang, “A Contrast Enhanced Ultrasound imaging system with diverging waves for cardiac imaging – initial evaluation on a beating phantom”
- Matthieu Toulemonde, Yuanwei Li, Shengtao Lin, Mairead Butler, William C Duncan, Vassilis Sboros, Robert Eckersley, Meng-Xing Tang, “Cardiac imaging with high frame rate contrast enhanced ultrasound: in-vivo demonstration”, IEEE IUS, Tours, 2016
- C.H. Leow, and M.X. Tang, “Spatio-Temporal Flow and Wall Shear Stress Mapping Based on Incoherent Ensemble-Correlation of Ultrafast Contrast Enhanced Ultrasound Images”, Ultrasound in Medicine & Biology, In Press, 2017
- Y Li, N Chahal, R Senior, MX Tang. Reproducible Computer Assisted Quantification of Myocardial Perfusion with Contrast Enhanced Ultrasound. Journal of the American Society of Echocardiography. (Under review)
- Y Li, N Chahal, R Senior, MX Tang. (2016) Segmentation of Myocardium in Contrast Echocardiography using Random Forest. 2016 IEEE 13th International Symposium on Biomedical Imaging (ISBI).